

Machine Learning &
Predictive Marketing
Lets first define Machine Learning (ML).
"Machine learning is a method of data analysis that assists with automating and improving analytical model building. It is a subset of artificial intelligence and based on the idea that systems can learn from data, identify patterns and make correct decisions with minimal human intervention. One big advantage is that a machine learning algorithm's accuracy can improve over time for less human effort."
The difference between Machine Learning (ML) and Artificial Intelligence (AI), is essentially that ML learns and predicts based on passive observations, whereas AI implies an agent interacting (online as an example in a chatbot) with the environment to learn and take actions that maximize its chance of successfully achieving its goals. Our EI Machine Learning algorithms takes customer data and turn it into actionable insights through accurate prediction of customer behaviour which enables specific targeted marketing to individuals. Machine Learning becomes more powerful with larger detailed data sets to learn from and at EI we have built very large robust training data sets for our ML optimisations. This is a big differentiator to other companies and contributes to our success.
​
Typical types of algorithms used in Machine Learning are: Linear Regression, Logistic Regression, K-Means and
Random Forest. The combination of the science of machine learning, statistics and the art of marketing is where we apply our skills and experience.
​
Predictive marketing is the use of machine learning models to help marketers anticipate future customer preferences and more accurately and timeously offer a relevant action. Mastering predictive marketing means more personalized and relevant experiences for customers and stronger, more valuable relationships for brands.
​
​
So how do we unlock the marketing Return on Investment with machine learning? See some examples below of how we use Machine Learning at EI.
​
1

Segmentation, Targeting & Personalisation
Key questions that marketers need definitive answers for, are:
-
Which customer segments are the most profitable and where do I focus my budget?
-
How do specific products perform within various customer profiles?
-
Which market segments are the best for launching the new products?
-
Which channels are the most profitable?
-
What opportunities exist to expand cross-category customer spending - up-sell and cross-sell?
-
Which customers should I include in my campaigns to maximize response rates?
​
Segmentation allows marketers to segment and profile customer and prospects which can yields gains of 25% to 150%. The below diagram depicts one type of segmentation strategies based on calculating only two values - the Customer Lifetime Value (LTV) and Propensity to Churn. This then informs the campaign tactics to use in order to maximise the value of the customer base. Within each major segment there will also be sub-segments that require different campaign treatments.​
​
​
​
​
​
​
​
​
​
​
​
​
​
​
​
​
​
Targeting the right customers with the right offers gives an additional gain of between 10% and 45% in response.
​
Personalization can deliver up to five times the Return on Investment (ROI) on marketing spend and lift sales by 10% or more.
​
When one combines all these techniques together through optimising and combining Machine Learning models, then you achieve a very powerful system that gains the best out of available budgets. Some of our ML combinations use up to 27 different models and without our experience of ML the optimisation across these different models would be very difficult due to competing "interests" between the models.
​
Some of the "output objectives" of the different ML models used in our ML system are:
-
Segmentation and clustering to group "like" people together using geodemographics and transaction data.
-
Response and conversion maximisation
-
Channel optimisation
-
Identification of market gaps and opportunities
-
Reporting and insights by persona, segments and cluster descriptions
​
Adding the right test techniques to the above "base system" to test each variable in the marketing mix then enables the maximisation of objectives. This assists customer value optimisation and also forms the base for effective and scalable customer acquisition strategies when matched with external market data.
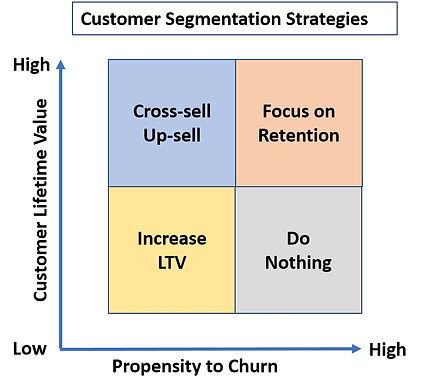
2

Customer Churn
The loss of some customers is inevitable. However as a company grows the size of their customer base it will attract some marginal customers who will tend to "churn" at a higher rate. If the customer acquisition strategy is more general and not sufficiently selective, then without careful management the churn rate will become a problem. It is well known that the cost of acquiring customers is a lot more than keeping a customer - often up to five times more - but many factors contribute to the real cost which is often much higher than forecast.
​
The typical cost groups associated with a high churn rate include:
-
Customer acquisition costs - Internal and external agent campaign costs.
-
Call centre costs
-
Retention campaign costs
​
The core problem underpinning the real costs is that as the customer base grows and more marginal customers are on-boarded, the costs of an increasing churn rate move from a linear to an exponential curve. This takes place as a company starts to maximise its "market share" of the Primary market customers and more Secondary or Tertiary market customers are acquired.
​
Therefore the first important step is categorizing each customer as to their future likelihood to cancel as it will then allow proactive management and treatment of the different "churn value" customer groups. Please see the segmentation diagram in (1) above. In addition if this is done in a manner that enables more selective customer acquisition strategies then this reduces the quantity of potential customers who will churn. The effective cost reduction of using the churn model to assist with customer acquisition more than doubles the ROI over a churn model used for internal customer management only.
​
We have built a proprietary framework of national data that our ML models run on. This allows us to build a churn ML model for a client and then to overlay it on our data framework. Once this is done then we can also assist with the customer acquisition aspects - to bring more of the primary market and less of the secondary and tertiary market potential customers.
3

Customer Lifetime Value (LTV)
Customer lifetime value is an important metric. It provides a picture of the business long-term and it enables year-on-year comparisons as to the quality of customers being onboarded and retained. High LTV is an indicator of product-market fit, brand loyalty and recurring revenue from existing customers.
​
The calculation of LTV measures the revenue and profitability of a customer over the "length of time" they stay as a customer. Naturally this needs to be measured for each "like" group and by acquisition channels and other pertinent variables. There needs to be a strict categorisation/rules of customer groups and the calculation process to ensure that comparisons over time are standardised and meaningful. Deviations across the entire customer base per annum can then be pro-actively managed operationally and all key metric changes explained to the board.
This is where Machine Learning models can play an important role in classifying the "like" groups and then measuring a number of key metrics to enable the active value management of the customer database. Once the models are built then their self-learning optimisation ability can maintain the models accuracy far longer than once-off built models. This is important as the LTV values should be measured quarterly to identify potential differences that could become problematic.
​
An example is when outbound acquisition campaigns are significantly changed and the overall response and conversion rates change by more than 10% in a particular market segment. In that case either more or less marginal customers may change the LTV, especially if there is a relatively high 1st year churn rate. Accurate and timeous measurement will then enable the most appropriate tactical strategies to be employed per problematic segment.